Unlocking the Power of Labeling Tool Machine Learning for Your Business
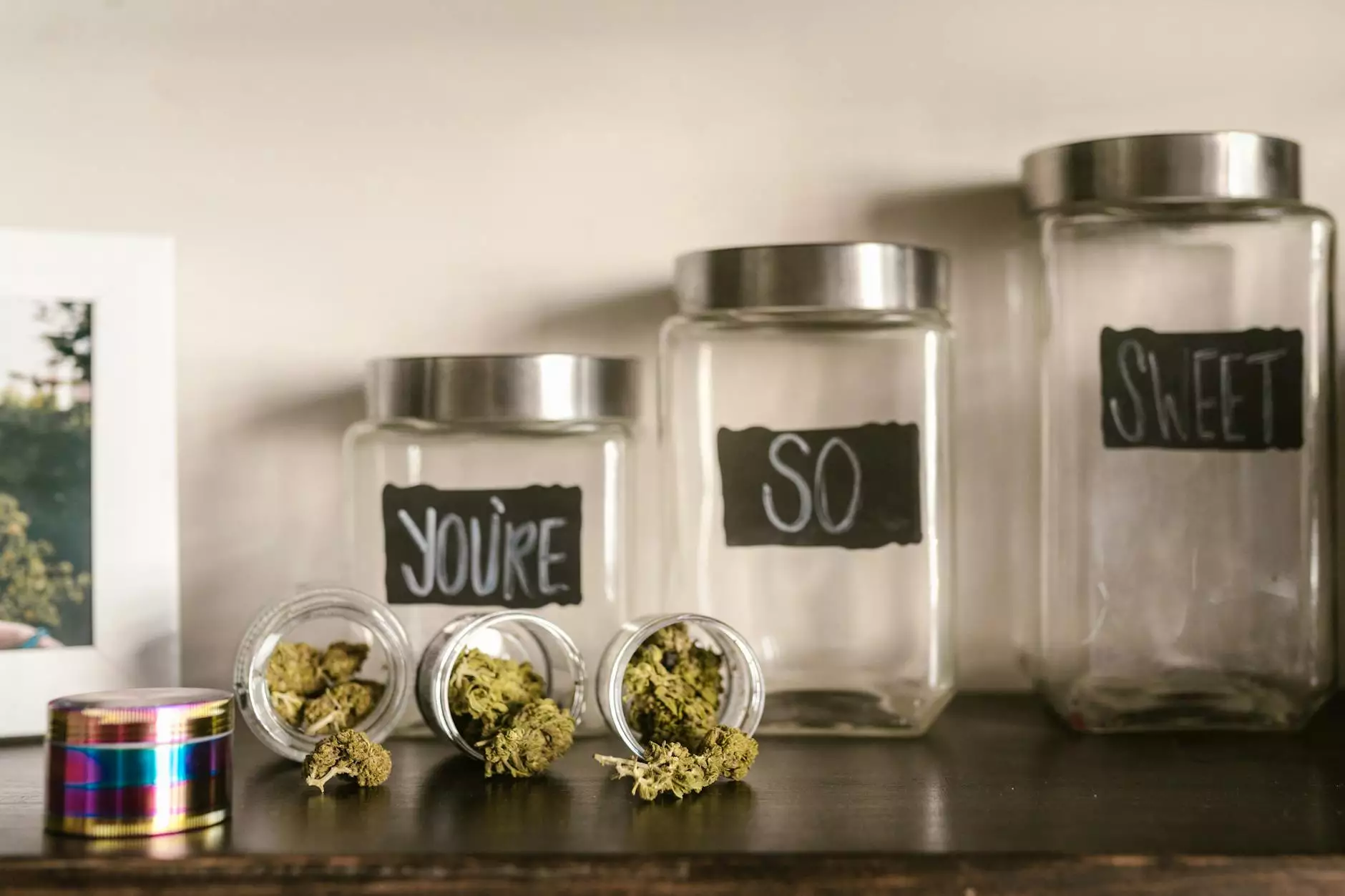
In today's data-driven world, businesses are increasingly recognizing the importance of high-quality data to fuel their machine learning models. At the heart of this data processing lies a crucial component: the labeling tool machine learning. This article delves into the significance of labeling tools, how they integrate with machine learning, and why choosing the right data annotation platform is essential for achieving robust performance in artificial intelligence (AI) applications.
What is Machine Learning Labeling?
Machine learning labeling is the process of annotating or tagging data so that it can be effectively understood by AI algorithms. These labels serve as a guide, helping models learn from the data by providing context and meaning. Whether you are dealing with images, text, videos, or audio, a premier labeling tool machine learning can streamline and enhance your data annotation processes dramatically.
The Importance of Data Annotation
Data annotation is the backbone of machine learning. Here are key points illustrating its importance:
- Machine Learning Accuracy: The quality of your model's output is only as good as the input it receives. Well-annotated data guarantees better predictions.
- Model Training: Data annotation allows machines to learn from the data, facilitating the creation of models that can make autonomous decisions.
- Scalability: Automated labeling tools increase the speed and scale at which data can be processed, vital for businesses with vast datasets.
Challenges in Data Annotation
Despite its importance, several challenges persist in the data annotation process:
- Resource Intensive: Data annotation can be time-consuming and require significant human effort, particularly for large datasets.
- Inconsistency: Variability in labeling quality can lead to unreliable datasets, negatively affecting model accuracy.
- Domain Expertise: Some applications require specialized knowledge, making it difficult to find the right annotators.
Overview of Labeling Tools and Their Capabilities
A labeling tool machine learning simplifies the data annotation process by offering several capabilities:
- Automated Tagging: Advanced labeling tools utilize AI and machine learning to automatically tag data, reducing the need for human labor.
- Collaborative Platforms: Many tools enable teams to work collaboratively, ensuring consistency and efficiency in the annotation process.
- Custom Labeling Options: Businesses can tailor labeling criteria to meet specific project needs, which enhances data relevance and accuracy.
Key Features to Look for in a Labeling Tool
When selecting a data annotation tool for your machine learning projects, consider the following features:
- User-Friendly Interface: A clear and intuitive UI allows for easier adoption and usage across your teams.
- Multi-Modal Support: Look for tools that support various data types, including images, videos, and text, ensuring versatility in applications.
- Integration Capabilities: The ability to integrate seamlessly with existing machine learning workflows or data pipelines boosts productivity.
- Quality Control Mechanisms: Implementing measures to evaluate labeling quality helps maintain high accuracy in your datasets.
Why Keylabs.ai Stands Out
Keylabs.ai offers an innovative solution in the realm of data annotation tools and platforms. Here’s why Keylabs.ai should be your go-to choice:
1. State-of-the-Art Technology
Keylabs.ai employs advanced machine learning algorithms to provide automated labeling features that enhance productivity and reduce time-consuming manual work.
2. Comprehensive Annotation Services
Providing a wide array of annotation services, Keylabs.ai meets the diverse needs of different industries, ensuring that every labeling task is handled with expertise.
3. Exceptional Customer Support
With a focus on customer satisfaction, Keylabs.ai provides excellent support, helping businesses navigate the complexities of data annotation.
4. Scalability and Flexibility
The platform is designed to scale with your business needs, making it an ideal solution for growing enterprises looking to harness the power of machine learning without compromising quality.
The Role of Labeling Tools in Different Industries
Across various sectors, labeling tool machine learning has diverse applications:
Healthcare
In the healthcare sector, accurate data annotation is crucial for diagnostics and predictive analytics. AI systems powered by annotated medical images can improve diagnostic accuracy and patient outcomes.
Automotive
The automotive industry employs labeling tools for autonomous vehicle training. Annotated video and sensor data are essential for developing self-driving technologies that rely on real-time data analysis.
Retail and E-commerce
In retail, labeling tools help with customer behavior prediction and inventory management. By analyzing customer interactions, businesses can optimize their sales strategies effectively.
Finance
In finance, annotated data aids in fraud detection by training models that identify unusual patterns in transaction data. This enhances security and minimizes risk.
Future Trends in Data Annotation
The future of data annotation is bright, characterized by exciting trends:
- Increased Automation: As technology advances, automation in labeling processes is expected to grow, leading to faster and more reliable results.
- Integration of AI and Human Efforts: Hybrid approaches that combine machine efficiency with human intuition will likely dominate, ensuring high-quality outcomes.
- Enhanced Data Privacy Measures: As data regulations tighten, labeling tools will need to incorporate stringent privacy measures to protect sensitive information.
Getting Started with Machine Learning Labeling
To kickstart your journey with a labeling tool machine learning, consider the following steps:
- Assess Your Needs: Clearly define what you want to achieve with data labeling and identify the types of data you will be working with.
- Choose the Right Tool: Based on the features discussed, select a labeling tool that aligns with your business needs and budget.
- Create a Plan: Develop a comprehensive data annotation plan that includes timelines, resources, and quality control procedures.
- Train Your Team: Ensure that your team is trained to use the annotation tool efficiently and understands the importance of quality labeling.
- Review and Iterate: Continuously evaluate the effectiveness of your data annotation strategies and be prepared to make adjustments as necessary.
Conclusion
The role of labeling tool machine learning in enhancing data quality cannot be overstated. With the right data annotation tool, businesses can unlock significant value from their datasets, leading to improved model performance and operational efficiency. Keylabs.ai stands ready to support your journey toward successful machine learning implementation, offering cutting-edge solutions that cater to your data annotation needs.
As the demand for high-quality annotated data continues to rise, investing in the right data annotation tool becomes essential. Embracing the future with tools like Keylabs.ai will empower your business to achieve new heights in AI and machine learning.