Unlocking the Potential of Agriculture with Machine Learning Datasets
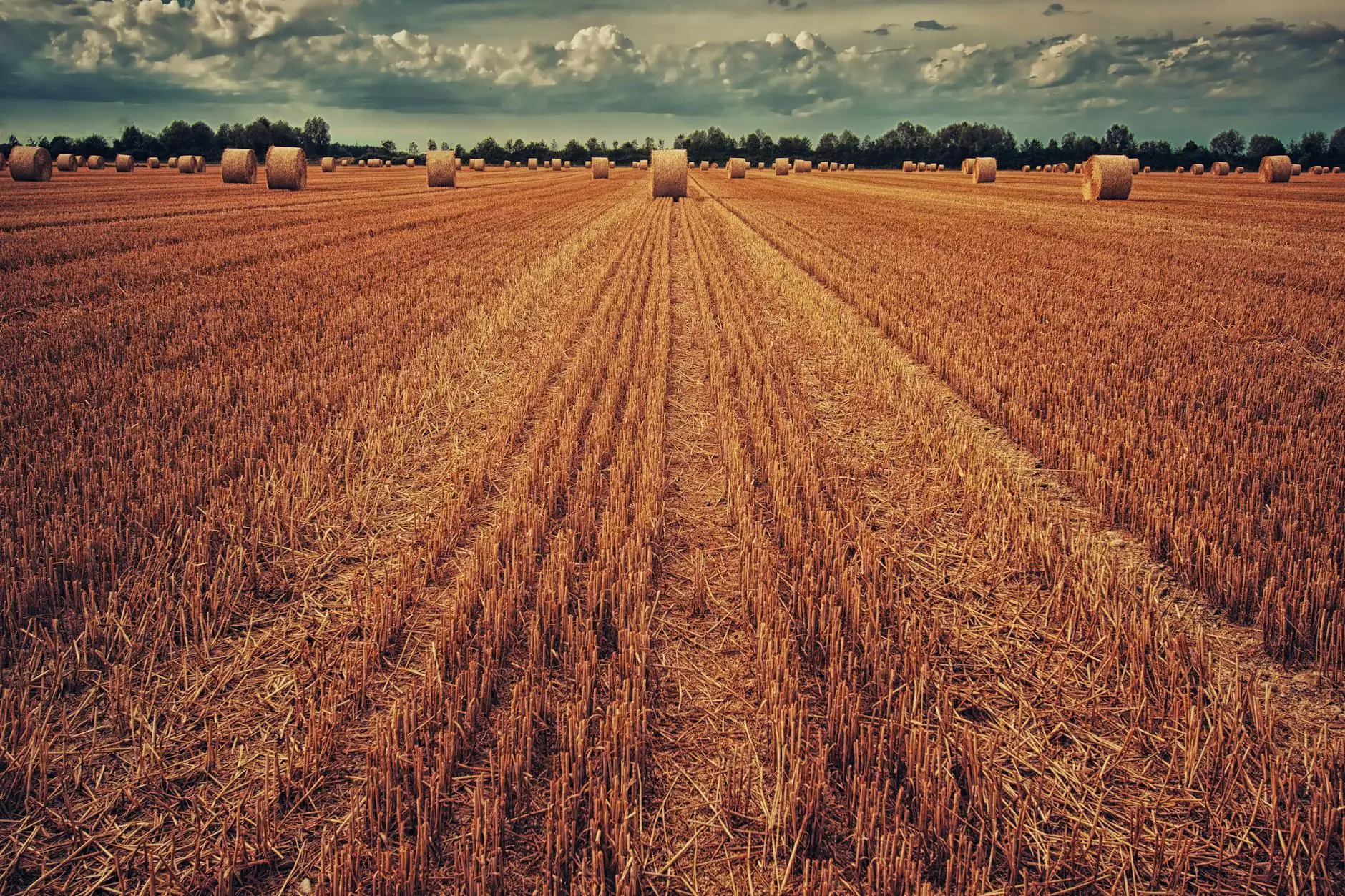
The agriculture industry is undergoing a revolution fueled by advanced technologies, and at the heart of this transformation is the integration of machine learning. In recent years, the utilization of agriculture datasets for machine learning has become increasingly pivotal for farmers, agronomists, and data scientists alike. These datasets, which comprise vast amounts of information regarding crop performance, weather patterns, soil health, and more, are essential for developing predictive models and intelligent systems that drive efficiency and sustainability in farming practices.
The Importance of Data in Modern Agriculture
Data is the driving force behind many innovations in the agriculture sector. Key reasons outlining its importance include:
- Optimizing Crop Yields: By analyzing data from various sources, farmers can make informed decisions about planting, irrigation, and fertilization, which directly leads to improved yields.
- Pest and Disease Management: Machine learning algorithms can predict pest infestations or disease outbreaks by analyzing historical data, enabling proactive measures.
- Resource Management: Enhanced data analytics allows for better management of water and nutrients, ensuring that resources are utilized efficiently and sustainably.
- Market Predictions: With access to comprehensive datasets, farmers can anticipate market trends and make more informed decisions about when and how much to sell.
What is an Agriculture Dataset for Machine Learning?
An agriculture dataset for machine learning refers to a collection of structured or unstructured data sets specifically curated for tasks in agriculture that can be analyzed using machine learning techniques. These datasets may contain various types of information, including but not limited to:
- Crop yield data
- Soil composition and health records
- Weather data (temperature, rainfall, humidity)
- Satellite imagery showing crop health
- Market prices and demand forecasts
The integration of these datasets into machine learning models can drastically improve various agricultural processes, leading to smarter farming and ultimately more sustainable practices.
Machine Learning Models in Agriculture
Machine learning models leverage the wealth of data at their disposal to learn patterns and make predictions. Here's how these models are being employed in various aspects of agriculture:
1. Predictive Analytics
With the help of historical data, farmers can utilize machine learning for predictive analytics to determine:
- The best planting dates based on weather patterns.
- Expected yields based on soil health and past performance.
- Potential pest threats and disease outbreaks.
2. Precision Agriculture
Precision agriculture involves using detailed data to manage field variability in crops. Through the use of agriculture datasets for machine learning, farmers can achieve:
- Targeted fertilization based on soil nutrient data.
- Optimized irrigation schedules based on precise weather forecasts.
- Reduced chemical use through smart pest management solutions.
3. Crop Monitoring
Modern farmers are turning to drones and satellite imagery to gather real-time data about crop health. By processing this data with machine learning algorithms, farmers can:
- Identify stressed areas in fields.
- Monitor growth patterns over time.
- Improve decision-making for planting and harvesting.
Benefits of Using Agriculture Datasets for Machine Learning
The advantages of utilizing agriculture datasets for machine learning are manifold. Here are some of the pivotal benefits:
- Enhanced Decision Making: Reliable data leads to better forecast models, helping farmers make informed decisions that can tangibly increase productivity.
- Sustainability: By optimizing resource use through data insights, agriculture becomes more sustainable, minimizing its ecological footprint.
- Cost Reduction: Efficient farming practices reduce waste and lower operational costs over time.
- Increased Profitability: Streamlined operations and smarter strategies lead to increased yield and better quality produce, ultimately contributing to higher profits.
Challenges in Implementing Machine Learning in Agriculture
Despite the numerous advantages, the integration of machine learning in agriculture does present certain challenges, such as:
- Data Quality: Ensuring the datasets are accurate, comprehensive, and updated regularly is crucial; poor quality data can lead to misleading results.
- Interoperability: There often exists a challenge in integrating different formats and sources of data into cohesive datasets for analysis.
- Cost of Technology: Investment in advanced technology and skilled personnel is required, which can be a barrier for smallholder farmers.
Case Studies: Successful Applications of Machine Learning in Agriculture
Now, let’s delve into some success stories where machine learning has made a significant positive impact on agriculture:
Case Study 1: Smart Irrigation Systems
A company focused on smart irrigation developed a machine learning model that analyzes data from soil moisture sensors and weather forecasts. By accurately determining the precise watering needs of crops, they reduced water consumption by 30%, allowing for not only cost savings for farmers but also conserving a vital resource.
Case Study 2: Crop Disease Prediction
In another initiative, researchers deployed machine learning models using historical data on crop health combined with environmental data to predict diseases in wheat crops. This model successfully identified disease outbreaks three weeks in advance, enabling farmers to take timely action and significantly decrease crop losses.
Case Study 3: Yield Prediction Models
Another innovative project developed yield prediction models by integrating satellite imagery and historical yield data. The insights garnered allowed farmers to adjust their techniques, leading to increased yields of over 15% in some regions.
How to Get Started with Machine Learning in Agriculture
For farmers looking to leverage the power of machine learning datasets in agriculture, consider these steps:
1. Identify Your Data Sources
Begin by compiling relevant datasets that can inform your specific decision-making processes. Look at sources such as:
- Local agricultural extension services.
- Government agricultural departments.
- Academic research publications.
- Commercial data providers.
2. Invest in Technology
Invest in the necessary software and hardware to analyze data. Consider cloud-based platforms that offer machine learning capabilities without the need for extensive local resources.
3. Collaborate with Experts
Engage with agronomists, data scientists, and machine learning experts to help you interpret data and implement actionable insights effectively.
4. Continuous Learning
Stay updated with the latest advancements in machine learning and agricultural technology. Attend workshops, webinars, and conferences to expand your knowledge and network.
Conclusion
As the world grapples with challenges related to food security, climate change, and resource depletion, the role of agriculture datasets for machine learning is more critical than ever. By embracing these data-driven technologies, farmers can unlock new levels of efficiency, sustainability, and productivity in their operations. The synergy between agriculture and machine learning not only presents a pathway to better farming practices but also paves the way for a future where data empowers every decision made in the field.
Embracing this revolution means ensuring that data is at the forefront of agricultural practices, enabling producers to grow more with less and ensuring that the agricultural sector can meet the demands of a growing global population sustainably.